Update history
Date | Version | Author | Update content |
---|---|---|---|
2024-12-31 | v1.0 | neucrack | Added source code, models, examples, and documentation |
Introduction
In certain applications requiring hand position or gesture detection, this algorithm can be utilized. It provides:
- Hand position with coordinates for four vertices.
- 3D coordinates of 21 hand keypoints, including depth estimation relative to the palm.
Example applications:
- Touch reading devices
- Gesture control
- Finger-based games
- Sign language translation
- Magic casting simulation
Sample image:
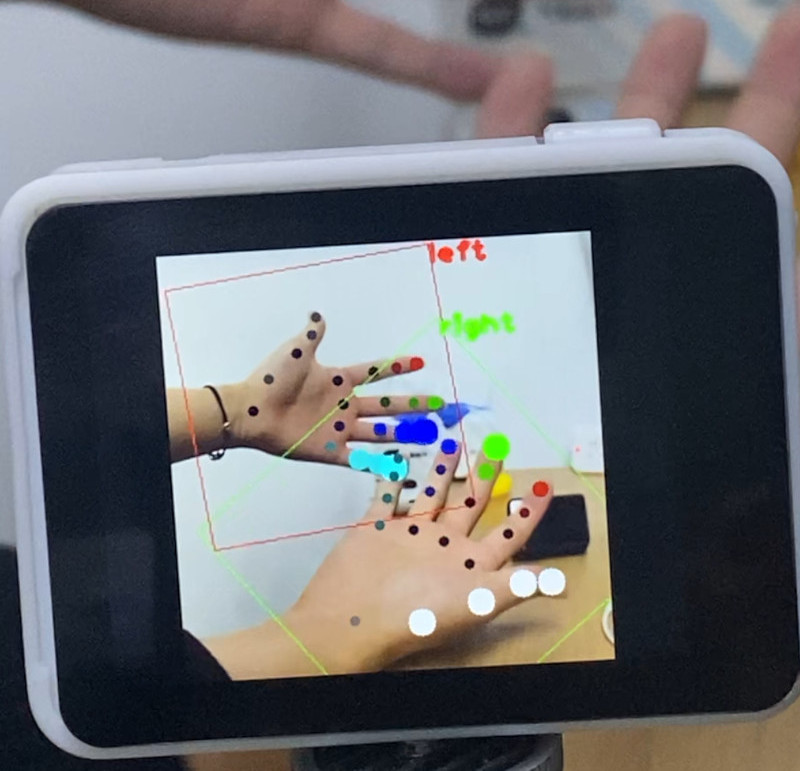
Sample video:
The 21 keypoints include:
Using Hand Keypoint Detection in MaixPy MaixCAM
The MaixPy platform integrates this algorithm (ported from MediaPipe for ease of use, firmware version >= 4.9.3 is required). The example can also be found in the MaixPy/examples directory:
from maix import camera, display, image, nn, app
detector = nn.HandLandmarks(model="/root/models/hand_landmarks.mud")
# detector = nn.HandLandmarks(model="/root/models/hand_landmarks_bf16.mud")
landmarks_rel = False
cam = camera.Camera(320, 224, detector.input_format())
disp = display.Display()
while not app.need_exit():
img = cam.read()
objs = detector.detect(img, conf_th = 0.7, iou_th = 0.45, conf_th2 = 0.8, landmarks_rel = landmarks_rel)
for obj in objs:
# img.draw_rect(obj.x, obj.y, obj.w, obj.h, color = image.COLOR_RED)
msg = f'{detector.labels[obj.class_id]}: {obj.score:.2f}'
img.draw_string(obj.points[0], obj.points[1], msg, color = image.COLOR_RED if obj.class_id == 0 else image.COLOR_GREEN, scale = 1.4, thickness = 2)
detector.draw_hand(img, obj.class_id, obj.points, 4, 10, box=True)
if landmarks_rel:
img.draw_rect(0, 0, detector.input_width(detect=False), detector.input_height(detect=False), color = image.COLOR_YELLOW)
for i in range(21):
x = obj.points[8 + 21*3 + i * 2]
y = obj.points[8 + 21** + i * 2 + 1]
img.draw_circle(x, y, 3, color = image.COLOR_YELLOW)
disp.show(img)
Detection results are visualized using the draw_hand
function. Keypoint data can be accessed via obj.points
, providing 4 + 21
points:
- The first 4 points are the bounding box corners in clockwise order:
topleft_x, topleft_y, topright_x, topright_y, bottomright_x, bottomright_y, bottomleft_x, bottomleft_y
. Values may be negative. - The remaining 21 points are keypoints in the format:
x0, y0, z0, x1, y1, z1, ..., x20, y20, z20
, wherez
represents depth relative to the palm and may also be negative.
Additionally, obj.x, y, w, h, angle
attributes provide the bounding box and rotation details.
Precision Optimization: The nn.HandLandmarks
class uses an int8
quantized model by default for faster detection. For higher precision, switch to the hand_landmarks_bf16.mud
model.
Relative Landmark Coordinates: By setting the landmarks_rel
parameter to True
, the function will output the 21 keypoints as relative coordinates to the top-left corner of the hand's bounding box. In this case, the last 21x2
values in obj.points
are arranged as x0, y0, x1, y1, ..., x20, y20
.
Advanced: Gesture Recognition Based on Keypoint Detection
Example: Rock-Paper-Scissors Detection
Two approaches:
- Traditional Method: Use code to classify gestures based on keypoint analysis.
- AI Model-Based Method: Train a classification model.
Approach 2:
This involves using the 21 keypoints as input for a classification model. Without image background interference, fewer data samples are needed for effective training.
Steps:
- Define gesture categories (e.g., rock, paper, scissors).
- Record keypoint data upon user input.
- Normalize keypoint coordinates to relative values (0 to object width
obj.w
) usinglandmarks_rel
parameter as described above. - Collect data for each category.
- Train a classification model (e.g., using MobileNetV2 in PyTorch).
- Convert the trained model to MaixCAM-supported format.
This approach requires knowledge of training and quantizing classification models.
Simplified Model Training Alternative
For users unfamiliar with PyTorch:
- Generate an image from the 21 keypoints (customize visualization).
- Upload the images to MaixHub.com for model training.
- Use the trained model in MaixPy for classification.
Complex Action Recognition
For actions requiring time-series analysis (e.g., circular motions):
- Store keypoint history in a queue for temporal analysis.
- Input historical sequences into a classification model for time-series gesture recognition.
- Alternatively, generate a single image from historical data and classify it.
These methods allow advanced gesture and action recognition leveraging MaixPy's integrated tools.
This version includes all details, including the explanation for landmarks_rel
.